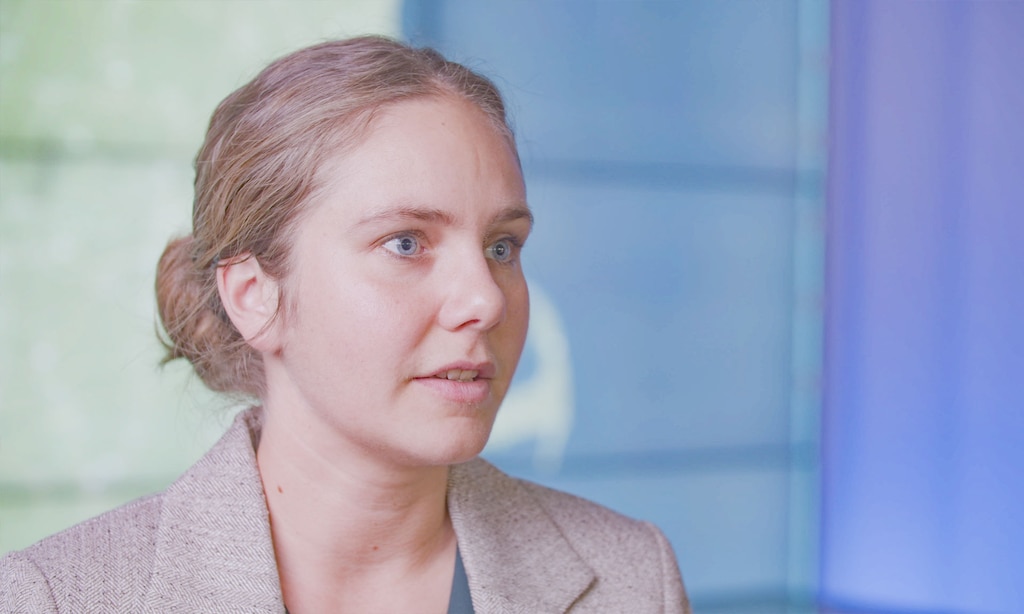
“Our systems will help businesses become future-proof”
Watch the video of the full interview >
About the research project
Optimising order distribution through the training of self-learning AI models is one of the goals of the research collaboration launched by the Massachusetts Institute of Technology (MIT) and Mecalux in 2024. Sarah Schaumann, from MIT’s Center for Transportation & Logistics (CTL), is the lead researcher of this initiative by the Intelligent Logistics Systems Lab, which focuses on prescriptive intelligence to help companies optimise the selection of shipping points for their goods.
Mecalux interviews Sarah Schaumann, Lead Researcher at MIT CTL, to learn more about the prescriptive intelligence project she leads as part of the MIT–Mecalux research collaboration.
-
What is the goal of the joint research project you’ll be leading with Mecalux?
This project aims to develop an orchestration model for distributed order management (DOM) systems based on machine learning. The idea is to replace the rule-based strategy commonly used today with an intelligent orchestration approach that leverages self-learning AI to assign orders to facilities and carriers. By using machine learning, we want to enable a system that’s not only able to improve operational efficiency but can also adjust to future changing environments over time.
The aim is to develop an orchestration model for DOM systems based on machine learning
-
What potential does this initiative have to shape the next generation of DOM systems?
We aim to build the future generations of smart and adaptable order orchestration strategies. By that, we mean replacing static rule-based approaches with intelligent, dynamic strategies capable of pivoting to changing customer demands, constraints or even market conditions. Ultimately, we want to set the stage for developing autonomous data-driven DOM systems that continuously self-learn. These are increasingly critical in dynamic environments.
-
How will you use reinforcement learning to develop optimal orchestration strategies?
In leveraging reinforcement learning, the model will learn and refine its orchestration processes by engaging with an environment. The model creates different orchestration strategies and gets a reward or a penalty depending on the result of the decision. It then adjusts the decisions iteratively. So, this is a continuous learning process where the rewards depend on the company’s business model. For example, one organisation might prioritise costs, while another focuses on delivery times.
We want to create intelligent, dynamic strategies capable of pivoting to changing customer demands, constraints or even market conditions
-
How is the Intelligent Logistics Systems Lab using simulations in this project?
Simulations allow us to replicate real-world scenarios in a safe and controlled environment. This means the model does not interact with the real system but with a simulated environment. This reduces the cost and the risk of testing and training those models. It also enables us to test their robustness and scalability more easily.
-
What impact do you expect this project to have in the logistics industry?
The environments in which companies operate are becoming dynamic and complex. The big advantage of learning-based models is that they adapt over time. This means that our systems will help businesses become future-proof.